Embedded Artificial Intelligence (AI) in Financial Services
IIS Executive Insights Cyber Expert: David Piesse, CRO, Cymar
“Despite the name artificial intelligence, there’s nothing artificial about it. As the industrial age amplified our arms and legs, the AI age is going to amplify our minds and our brains”. Manoj Saxena Executive Chairman of the Responsible AI Institute
This erudite quote encapsulates using AI to boost human insights and creativity. During the pandemic doctors placed expert decision making into AI datasets helping nurses deal with stress situations[i].Businesses turn to AI for first mover advantage, competitive edge, future of work, mundane business process automation, advanced analytics and human augmentation. Challenges abound around ethics, privacy, liability, cybersecurity, bias, transparency and loss of job. AI removes repetitive tasks so employees that learn, will prosper as new jobs are created. Successful adoption centres around data integrity, verified trust and acting responsibly with exponential technology. Until recently AI had a relatively narrow focus looking at expert systems and performing singular tasks. Vendors competed for the best algorithmic approach with future promises of AI still in research labs and a perceived endgame in science fiction realms. Digitisation increased data volume, smart devices and systemic cyber risk bringing more AI technology out of the labs into production. Practitioners call this “third wave AI“ and this paper looks at the implications of this paradigm shift on financial services moving from a pure algorithmic to a data driven approach as industries start to value data at the boardroom level. This innovation is recognised in global investment sectors where digitisation and AI lead the investment themes followed by renewable energy and cyber security both of which are becoming powered by AI. The stage is set. The following diagram show the AI development over a timeline.
The first AI wave, took an expert system approach by gathering information from subject domain specialists and ingesting experience based rules. The second AI wave was driven by machine learning techniques to counteract risks of climate change, cybersecurity, digitization outcomes and better modelling results. Models were trained to predict outcomes but did not address uncertainty, being based on probability theory, Monte Carlo simulations[ii], and essentially backward looking. The relevance of AI to spreadsheets became clear, as millions of users with modeling solutions, extract data from spreadsheets to run random simulations stochastically to give probability and correlation of risk across enterprises. The result is a combination of first and second wave AI into workable, but not fully dependable, statistical models, that in general lack forward looking and predictive capabilities. Moving to the third wave machine learning datasets build transparent, underlying explanatory models with perception of uncertainty, using real-world scenarios with real time data. This co-pilot approach augments human creativity with machine learning and has implications for all industries. The insurance industry sits in the cross hairs for augmentation at the underwriting stage to better identify risk to appropriately price premiums and at the claims stage, reducing fraud and improving loss ratios by enabling more competitive pricing, resulting in faster parametric claim payments for customers. AI helps stock exchanges by tracking markets with clarity and understanding, in real time, without delay or distortion, bringing boards and investors closer together. Banks get a better approach to credit analysis. All financial services benefit from customer experience, efficiency and also challenged by the same risk register.
The articulation of “Causal AI” has the means to change models to utilise causality over correlation, explaining decisions by cause and effect. Causality has evaded AI progress to date and is the advancement of contextual third wave machine learning by unlocking black boxes and explaining decisions to accountable third parties such as regulators, expert witness courts, forensics, lawyers and auditors. Causal AI needs to be embedded in models and spreadsheets to get transparency. The insurance industry has recognized causality for time immemorial but represented it mathematically by correlation, a statistical method measuring dependency of two variables. Rational counterfactual explanations require machine learning and underlying causal models taking a contextual premise beyond statistical associations.
Until recently the great academic AI debate has been about Artificial General Intelligence (AGI), where machines start thinking like humans and how this intelligence leads to training machines to create new tasks versus Artificial Super Intelligence (ASI), a consensus of AI exceeding human cognition by total automation. The crux of the debate gets back to regulation, ethics and responsible AI so likely the AGI proposition will hold true as although AI self-awareness will emerge in machines, humans will remain in the loop as expected.
Emerging popularity of Generative AI (GenAI) third wave tools, like Open AI ChatGPT[iii], portend to expand creativity and eventually enhance predictive modelling. AI is an umbrella term and detractors are saying that GenAI tools are not fully AI but acts like a stochastic parrot[iv] as they are trained on volumes of data that are pre-generated and require continuous human input for mainstream adoption. The debates rage on, but announcements by Microsoft at WEF Davos[v] to build GenAI into apps and dashboards is a significant accelerator. If these tools are embedded in power-point or spreadsheet cells then transparency and causality are ingrained in extant everyday work tools. This opens up a new dimension in the cloud computing and AI search engine race which has nudged Google to create equivalents. GenAI impact will help finance boards make informed investment decisions. By training these tools on financial data, companies leverage machine learning capabilities, identifying trends and patterns invisible to humans. It will take time before data sharing challenges allow all this data to be ingested as many regulations surround the use of data sharing, privacy and AI needs an established code of conduct manifesto.
While ChatGPT may not replace the need for all human financial advice, at least in the near future. It may turbocharge service providers' ability to provide trusted advice and planning on the full range of financial services – ETBFSI.com[vi]
AI changes the landscape of cybersecurity by detection speed and predictive response to emerging threats, improving security. Conversely GenAI can create malware scripts by bad actors for malicious purposes posing a risk to cybersecurity posture. Cyber risk needs to be mitigated at the design stage and cyber integrity maintained at all times as data used for training today will be the basis of machine learning in the future. This has to be done now, not added on, as discovery will be too late as AGI emerges and control of data could be lost.
Enterprise AI can be centralized (more risky), or decentralized with blockchain integration, revolving around data integrity (preferred). Transformation by machine learning is a boardroom issue. Complete enterprise AI solutions are emerging as low code no code, API[vii] driven software to help companies leapfrog in AI whilst also addressing issues of reputational risk, solvency, brand awareness, operational resilience and data accuracy. AI regulation is a sensitive matter as algorithms shown to regulators reveal secret sauce IP. Technology itself is not regulated but instead the models, business processes and data flow that leads to customer outcomes, societal impact and bias assessment. The use of multi modal AI which holistically covers many media types (video, text and images), the reduction in social media deep-faking, embedding of AI software into hardware chips and use of synthetic data to train machines to avoid breaking privacy laws are enterprise developments that are evolving in the short term requiring corporate board governance, oversight and direction.
The 4th Industrial Revolution[viii] is realised with Web3, Metaverse and AI. Blockchain protects the data layer and must underpin all data accessed by AI. Internet, cloud computing , mobility and blockchain have taken years to be adopted but the embedding of AI is just taking months. This explosion of data cum intelligence will shorten the time for quantum computing and causing the post quantum[ix] standards (for encryption continuity) to accelerate. The mainstream adoption of the metaverse in parallel, integration of AI into avatars and NFT’s impacts evolution of decentralized finance[x] (DeFi) and the future of cryptocurrency. All these trends were alluded to at the WEF 2023. Ultimately it is about ensuring each piece of data has situational awareness. This is not a short term issue about trusting third wave machine learning systems but a long term issue as outputs are created on which future systems will be trained so data standards must be mandatory otherwise we risk living in an ocean of false information sources and misleading data. Now is the time to act.
Financial services adopting third wave explainable AI will achieve transparency by evidence based data decision-making and de-risk AI adoption fears, with human input at each stage of the value chain to give confidence systems are operating correctly. This drives transformation from a business outcome perspective with governance to stop automating bias at scale. Trust is maintained with full transparent explanation, cyber integrity, compliance to privacy/AI accountability laws thus mitigating residual risk from implementation and avoiding fines or reputational risk. AI implementation will require a holistic approach by companies led from the top avoiding compliance silos. Like cyber, AI integrity needs to be by design, to get operational assurance using AI maturity models[xi], NIST[xii] standards and certifications.
What of AI trends and the future? Sensors are a large part of the industrial world with consumer devices increasing to 80 billion by 2025[xiii]. The convergence of IOT and robotics change how humans interact in the real world. Autonomous transport, drones, smart agriculture equipment, surgical robots and 3-D-printed buildings will become pervasive forcing open-source data sharing standards. Wearable device data can be ported directly to insurers, smart home and auto data made available through cloud computing so consumer device manufacturers can embed insurance at the OEM level. Public private partnerships will help create a common regulatory and cybersecurity framework around the AI paradigm.
“Artificial intelligence stirs our highest ambitions and deepest fears like few other technologies. It’s as if every gleaming and Promethean promise of machines able to perform tasks at speeds and with skills of which we can only dream carries with it a countervailing nightmare of human displacement and obsolescence. But despite recent A.I. breakthroughs in previously human-dominated realms of language and visual art — the prose compositions of the GPT-3 language model and visual creations of the DALL-E 2 system have drawn intense interest — our gravest concerns should probably be tempered”.
Yejin Choi, a 2022 recipient of the prestigious MacArthur “genius” grant
Definitions
Artificial intelligence is a type of intelligence displayed by machines, as opposed to the natural intelligence displayed by humans and other animals. AI is an entire branch of computer science and the term is often used interchangeably with its components.
Source: Toolbox https://www.spiceworks.com/tech/artificial-intelligence/articles/what-is-ai/
Auto-Imputation AI is a method to add missing data based on knowledge using random distribution to avoid bias.
Causal AI is an AI system that explains cause and effect in decision making
Cognitive Computing is the simulation of human thought to help solve real world problems.
Computer Vision trains computers to capture and interpret information from video and image data.
Counterfactual Analysis interrogates a predictive model to determine what changes to a data point would change the outcome (cause and effect). Often known as artificial imagination.
Deep Learning is a subset of machine learning utilising neural networks which mimic the learning process of the human brain based on artificial neurons connected together representing relationships between data.
Feature Sets in machine learning are measurable data parameters relating to an event.
Fuzzy Logic is how computers deal with uncertainty and reasoning being used by generative and third wave AI to reason on cause and effect relationship (counterfactuals).
Generative AI is AI used to create new text, images, video, audio, code or synthetic data and are also known as Generative Pre-Trained Transformer (GPT).
In Context Learning is ongoing research linking machine learning to the real world.
IOT is the Internet of Things and convergence of devices connected to the Internet.
Machine Learning is a technique giving computers potential to learn without programming. It enables machines to identify patterns ,translate, execute and investigate data for solving real-world problems. Training can be done as supervised learning with actual classified datasets or unsupervised with computer generated synthetic data ,anonymised from real world datasets. Supervised Learning builds predictive models based on past examples.
Natural Language Processing (NLP) is the interaction between computers and human language analysing large amounts of language data leading to large language models (LLM) such as ChatGPT.
Privacy Enhancing Technologies are tools maximising use of data while preserving privacy.
Reinforcement Learning is the ability for a computer to determine from an algorithm what action to take to create a new task without adding further training data.
Responsible AI is the practice of designing, developing, and deploying AI with good and fair intentions to empower employees, customers, businesses and society, engendering trust and ability to scale AI with confidence without bias.
Robotic Process Automation is software robotics where repetitive tasks are done quickly and accurately by machine. Not to be confused with Robotics which is an interdisciplinary field of science and engineering incorporated with mechanical engineering, electrical engineering and computer science.
The Three Waves of AI
AI has been defined by DARPA[xiv], MIT[xv] and other practitioners as having evolved over time in three distinct waves. The first wave took information and experience from subject domain experts, capturing rules without creativity, learning or addressing uncertainty. Progress made in cybersecurity and climate change risk mitigation plus digitization outcomes such as autonomous transport led to the second wave which involved machine learning, biometrics (face and voice recognition) utilising probability theory and Monte Carlo simulations. It was realised that “big data” needed to be classified into smaller datasets that can learn and adapt to situations albeit with limited capability to abstract knowledge from one domain and apply it to another. Second wave have capabilities to classify and predict the consequences but do not understand “why” in context or utilize reasoning. Integrating AI to spreadsheets becomes obvious as current models use cells to run statistical simulations using probability and correlation which in many cases is looking at events in the rear-view mirror.
Third wave uses machine learning datasets to build underlying explanatory models using counterfactuals, allowing them to characterize real-world scenarios. Whereas second wave AI systems needs vast amounts of (re)training data, the third wave strives to create new tasks without being retrained. These models are contextual and collaborative with humans giving relevant responses, accurate predictions and making decisions abstracting knowledge data for further use. The two waves that form the current AI status quo by themselves are not going to be sufficient hence the need to bring them together and commence the third wave around contextual adaptation as shown in the DARPA diagram.
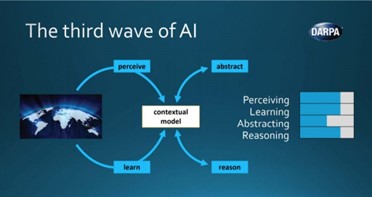
Source: DARPA https://blogs.cardiff.ac.uk/ai-robotics/an-excellent-talk-on-the-three-waves-of-artificial-intelligence-ai-the-contextual-adaptation-is-a-way-to-go-explainable-ai/
Data driven approach in machine learning training is vital to good model outcome in comparison to a pure algorithm technique. Companies have raw data from sensors, enterprise data warehouses, analytic model output and these sources are pulled into model engineering. Before this data is ingested it needs to be transformed smaller classified datasets which become the predictive variables for the target sector. With deep knowledge of the data, missing values can be added by auto-imputation and data cleansing.
Explainable XI (XAI)
The capitalized value of organizations is achieved by leveraging intellectual capital, capturing knowledge, storing it and make available for reuse. An example in insurance would be to capture knowledge about data parameters on rating and pricing for a line of business in order to get a more accurate pricing model which can then train underwriters and provide explanation to customers. Additional knowledge is captured by creating feature sets which are small measurable datasets containing details of specific line of business such as marine , construction etc. When these parameters change from internal/external influences the rating table moves. Feature sets are essential inputs to enterprise critical processes including reporting, forecasting, planning, optimization and machine learning and once created can be used in applications such as underwriting, bindable quotes, price forecasting and modelling. Domain expertise creates feature sets as human augmentation selects the best decision to get to the right outcome. Organizations that have not captured knowledge suffer loss of capitalized value. Capturing and storing knowledge in the form of feature sets significantly improves the outcomes of subsequent processing such as forecasting and integrated business planning to generate an annuity revenue stream. Base knowledge is captured but third wave AI also captures causal knowledge which is the structure of counterfactual dependence among multiple variables. This is vital to an industry or company that needs to explain decisions to interested third parties moving away from a black box and this leads to explainable AI (XAI) as clearly illustrated by the DARPA diagram below.
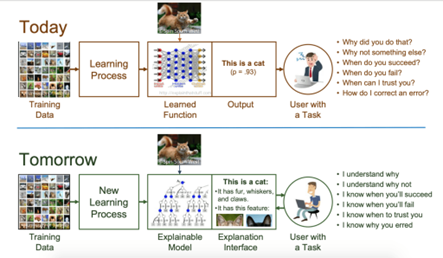
Source: DARPA https://www.researchgate.net/figure/Explainable-AI-XAI-Concept-presented-by-DARPA-Image-courtesy-of-DARPA-XAI-Program_fig12_336131051
Explainable AI is achieved by formal hypothesis testing which means testing a relationship between two or more variables with a proposed evidence based explanation for outcome of an extraordinary event. This is an important aspect of data science testing and needs to ingest previous observations. If you do not like the outcome then what can be done in a predictive manner to address the management of that risk over space and time adding reasoning to AI learning and training. Explainable AI is a set of tools and frameworks to help humans understand and interpret predictions made by machine learning models.
AI and Causality (Causal AI)
Causality is needed [xvi]to unlock real progress in AI and necessary to move to the third wave progress of machine learning. Legacy AI systems are black boxes, not aligned with human values and no explanation capability. Gartner in 2022 listed Causal AI as a key emerging technology and innovation trigger, transforming businesses where early adopters can achieve significant benefits.
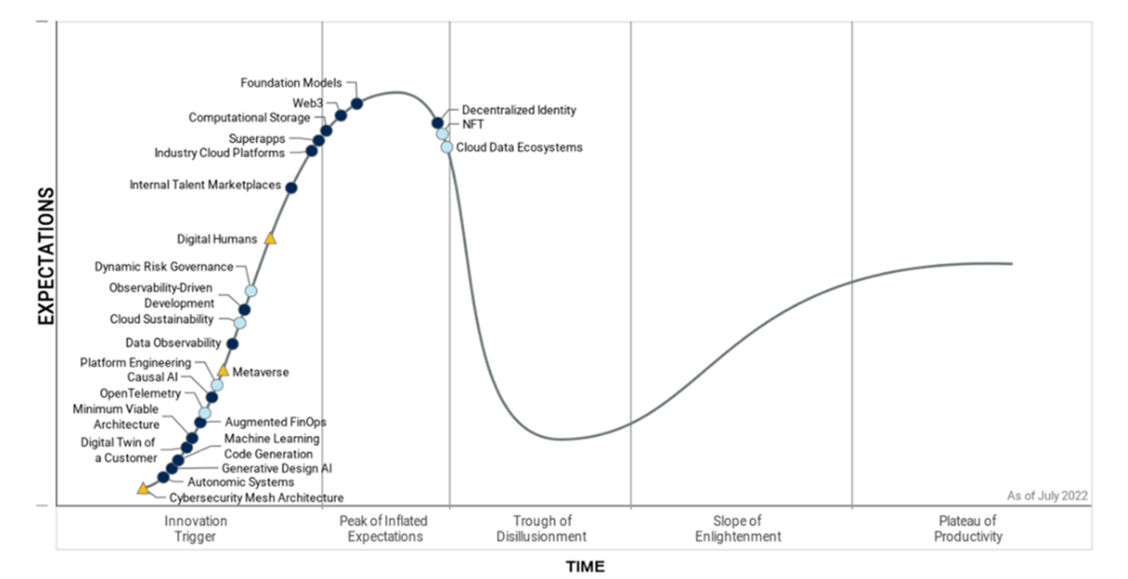
Source: GARTNER https://www.causalens.com/gartner-lists-causal-ai-as-a-flagship-emerging-technology/
Models embedding causality identify critical information in datasets, discarding irrelevant or outlier correlations improving predictions. Humans rely on causal reasoning to diagnose cause and effect and AI augmentation helps anticipate how actions will impact outcomes. Causal AI introduces “explainable AI” into the third wave creating an underlying causal inference model going beyond statistical associations between variables. If capital markets, for example, leverage causality in new AI investment analysis strategies this can create alpha by identifying new causal relationships in economic, financial and alternative data. Causal AI sparks counterfactual analysis debates on causation vs correlation in predictive modelling. A counterfactual explanation describes the predicted outcome of an event, the causes and values that were input to the model that caused a certain prediction. AI can report and evaluate all counterfactual explanations and state how it selected the best one or generate multiple diverse explanations, so a regulator gets access to multiple viable ways of generating a different outcome to show fairness and unbiased approach to pricing or other scenarios.
Correlation is a statistical association that measures increasing or decreasing trends between two variables. Causality is the empirical relation between two events or variables such that a change in one (the cause) brings about a change in the other (the effect). Causation in AI is preferred to correlation because it explains why two variables interact in a predictable and rational manner. Establishing causation is more difficult than establishing correlation hence a need to use AI. Correlation analysis is purely statistical in nature and can be managed through high performance computing power. Causation analysis, on the other hand, requires more than just computing power as rational explanation is a plausible narrative connecting the variables to real world circumstances or behaviours that contribute to the risk. Eumonics[xvii] are developing AI platforms based on knowledge capture, causality and third wave AI.
The Artificial General Intelligence Debate (AGI)[xviii]
AI is arguably more transformative than the industrial age leading to impactful social changes or consequences by creating new things, interacting with uncertainty whilst learning in parallel in a non-linear fashion. With a topic so transformative or controversial in nature it is worth looking at the AGI debate which discusses reaching a stage where machines start to get human level intelligence, think like humans and become self-aware using reasoning to create new tasks and scaling up AI programs with humans in the loop. The other side of the debate is Artificial Super Intelligence (ASI)where the world becomes fully automated and that is out of scope for this paper. The practitioner view is that AGI will happen in the next decade
Large language models[xix] (LLM), trained on large amounts of real-world data will assist AGI development as they mature, but currently they have no world model from which to draw conclusions. The use of reinforcement learning to create multiple forward paths, reasoning with explanations using simulations is a key driver to AGI and needs to be layered above the large language models. Currently AI fails to address human cognition which is why causality and counterfactuals are key. The AGI stage will potentially replace more than 50% of current human work once reasoning is simulated and AI limitations are removed, but new jobs will be created so education for all in AI is fundamental. Governments must innovate and be visionary to meet the challenges and opportunities that the development of AGI presents even though the time horizon is uncertain. Regulation and ethics leading to Responsible AI is best managed using reinforcement learning combined with human feedback. Like any technology risks increase when AI is used by bad actors, especially as LLM’s become easier to train.
Transparency and explainable AI are critical but the human augmentation avoids bad decisions. For example, complex parametric insurance claims even though handled by AI and blockchain smart contracts require a human intervention stage to ensure that basis risk is managed and claims are handled correctly. Simple claims can be automated. Augmenting humans with AI has greater economic benefit than just replacing human labour. Humans must learn AI and AI must understand humans. A good reference to this debate is The Age of AI - Our Human Future[xx], written by Henry Kissinger, Eric Schmidt and Daniel Huttenlocher, discussing human AI involvement over dominance of machines and most recently updated by an article of the same authors in the Wall Street Journal[xxi].
Generative AI (GenAI)
GenAI is not new but LLM’s have improved exponentially by creating images, text and writing new content with human augmentation. Emergence of OpenAI and Stability AI[xxii] in 2022 and announcements in WEF Davos 2023, where generative models like ChatGPT were made available at an affordable price or in an open-source environment, has changed the playing field. Seed investors are backing generative AI companies as they see large valuations once they graduate to the Series A stage but fears arise about it becoming a commodity as the tools become easier to train. There is a large ecosystem of generative AI companies as shown below.
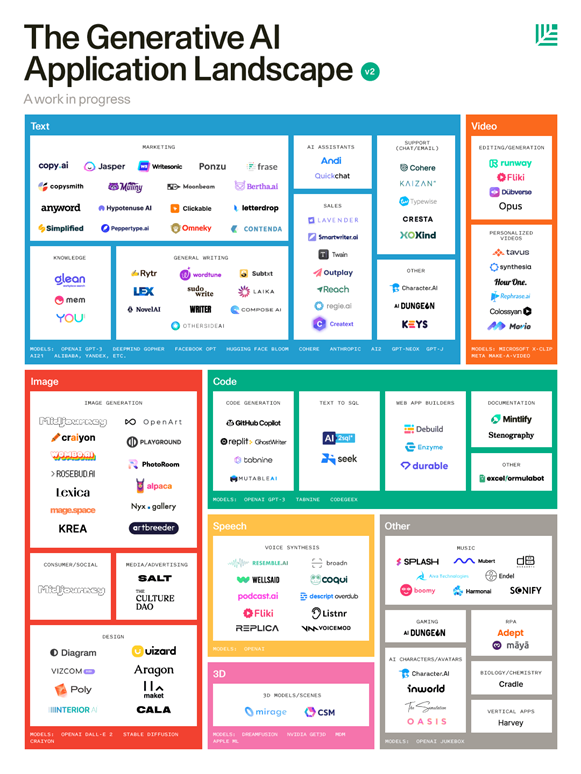
Source: https://www.protocol.com/generative-ai-startup-landscape-map
OpenAI was the market leader as of 2022 as shown in the chart with $1B invested and ChatGPT amassing over 266 million total users since its launch in November 2022[xxiii].
GenAI tools can write blockchain smart contracts which can be turned into Non Fungible Tokens (NFT’s). This means they can become chatbots for the crypto world. ChatGPT is currently only trained on data to 2021 and the full impact will be seen once it is fed with more real time data. GenAI becomes evolutionary when it is infused in apps and infrastructure so PowerPoint can receive requests to self-populate decks or using dashboards with surface patterns and metrics within EXCEL spreadsheets. This will enable tracking predicting and optimising behaviour more efficiently. In an unprecedented flip AI becomes the creator of content and humans becomes the custodians or curators. It is early days and this graphic explains where we are now but growth is exponential and not linear.
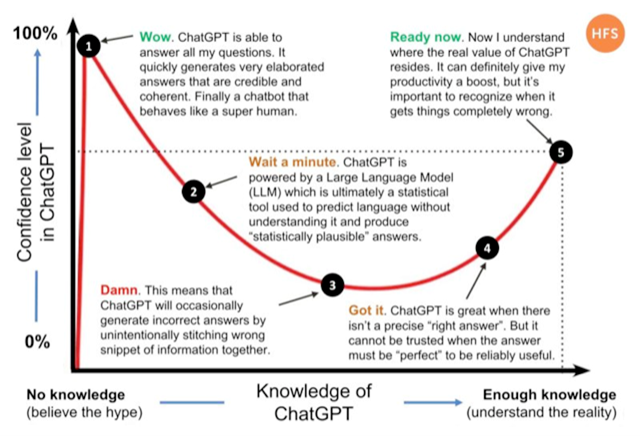
Source: HFS RESEARCH https://re.linkedin.com/posts/bertalanmesko_hfs-research-shared-this-useful-graph-about-activity-7028709074149994498-N2j3
GenAI paradigm is capable of changing financial services by analysing large data volumes, dealing with complex financial queries and provide personalized recommendations using conversational AI thereby supercharging existing robo-advisor methods boasting $2.67 Trillion AUM[xxiv] including investments, life insurance, pensions and financial planning.
GenAI is premised on the use of neural networks, a form of machine learning in which millions of interconnected processing nodes extract a predictive function by analysing underlying causal relationships in a set of data. LLM’s like OpenAI’s GPT-3 are leviathan neural networks that can generate human-like text and programming code. Trained on internet data and retrained to learn new tasks, these models can evolve to in-context learning[xxv] where new tasks can be accomplished without retraining which is a step towards AGI by a transformer model. Ongoing research on in-context learning shows these models contain smaller linear models which the large model can leverage to implement learning algorithms to train the smaller model to complete new tasks with information already contained within the larger model. Intelligent Data Processing (IDP) can process documents without human intervention, improving automation and accuracy of data extraction using OCR (Optical Character Reading). LLM’s assist with unstructured data by ingesting OCR feeds and keep humans in the loop by elevating them in the value chain to perform higher functions such as cross selling and tailored customer experiences.
AI and Cybersecurity
AI helps organisations reduce risk of data breach, improve overall security posture by learning to identify patterns from past data to make predictions about future attacks. AI powered systems can be configured to automatically respond to cyber threats in real time. As the corporate attack surface increases analysing cyber threats is no longer a human-scale challenge. Neural networks are required to augment security teams to assess cyber risk and deal with the billions of time-dependent signals and threat intelligence emanating from Security Operation Centres (SOC). Financial services, like mobile banking, have created new vulnerabilities through convergence of banking, telecom, and information technology requiring implementation of advanced cybersecurity protocols. such as encryption, blockchain, firewalls, and multifactor authentication. By capturing expert knowledge on cyber-attacks AI can be used to train employees in cybersecurity practices to mitigate the human error risk, which is a high percentage of cyber risk, as AI performs repetitive activities with flawless capability. This is vital as critical infrastructure moves to the cloud.
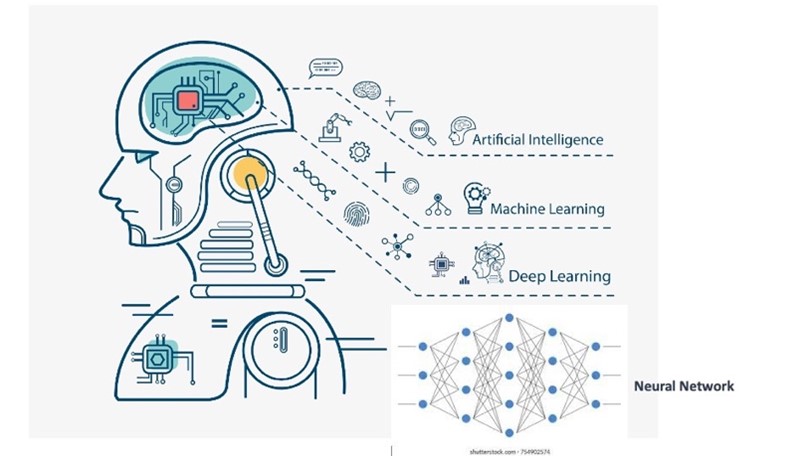
Source: https://www.saasworthy.com/blog/artificial-intelligence-and-machine-learning-in-cyber-security/
Machine learning and hyper-automation detect anomalies and unexpected network activity using deep learning to train a neural network to automatically classify data into malware categories that can be dealt with efficiently, reducing false positives. Explainable AI provides justification for these decisions. On the flipside cybercriminals can train AI systems to carry out large-scale attacks. GenAI tools can generate malware that could be used for ransomware extortion. Natural language processing can collect predictive intelligence by scraping information on cyber dangers and curating material. AI-based security solutions can provide neoteric knowledge of global and industry-specific threats, allowing more informed prioritising decisions based on what is most plausible to attack infrastructure and data. Deepfake images plague social media and AI can develop deepfake detection platforms using blockchain for photo and video identification via cryptographic seals around images. This allows reverse engineering to uncover the source and detect attributes used to create the deepfake. With advancements in quantum technologies, there is additional risk in the security space. NIST has introduced new standards for post-quantum cryptography and replacing existing PKI/RSA cryptography[xxvii] to ensure privacy and confidentiality continues unabated.
AI and Regulation
AI systems will alter the way governments operate on a global scale and it is critical to understand current and proposed legal frameworks. Differing standards are emerging from China, European Union (EU), and USA. It is prudent for regulators to avoid overburdening businesses to ensure they leverage AI benefits cost-effectively. In 2022, China passed regulations governing use of AI algorithms in online website suggestions mandating complete transparency with opt out clauses. European Commission (EU) passed the Artificial Intelligence Act[xxvii] categorising AI services such as low risk, high risk, or unacceptable risk, applying corresponding obligations depending on the risk level. Registration of high-risk AI systems, such as remote biometric identification systems, are required with implementation in second half of 2024, potential non-compliance fines will be 2-6% of company annual revenue akin to General Data Protection Regulation (GDPR)[xxviii] which already has privacy implications for AI use. In USA AI regulation is being imposed at state level. Nationally in 2021 the U.S. Congress enacted the National AI Initiative Act[xxix], an umbrella framework to strengthen and coordinate AI research across government departments and a year later the Algorithmic Accountability Act[xxx] dealing with impact assessments from AI systems leading to biased outcomes. National Institute of Standards and Technology (NIST)[xxxi], is working to develop trustworthy AI systems based on third wave for transparency, fairness and responsibility including AI regulatory sandboxes establishing a controlled environment for the development, testing and validation of start-up solutions in real world conditions. Islamic countries believe AI should be designed to “do no harm”, calling for greater regulation of the technology under Shariah Law. That means there two billion people who do not yet have clear regulation on AI or what is Islamically permissible (Falah)[xxxii] and what outcomes it should deliver. Whatever the country the result is consistent in acting responsibly with AI and in the USA the Responsible AI Institute[xxxiii] has been created for this purpose.
AI and Financial Services
AI impact on financial services is transformative improving personalized customer experiences and operational efficiency. With AI-powered chatbots and virtual assistants, 24/7 customer support can be provided. Personalized financial advice can predict financial needs, enabling tailored services and products while at the same time de-risking cybercrime, bias and building risk management initiatives by design. Integrating AI with complex, fragmented legacy systems can be challenging, so an integration plan is required making sure employees have the right understanding and skills. Model governance is required to detect early dataset changes creating collaboration between data science and business teams leading to shared financial dashboards. Bayesian modelling[xxxiv] for predictive modelling capability was used in the pandemic to perform better on sparse prior knowledge data which is lacking in many intangible emerging risks. This gives model confidence to measure uncertainty giving highly interpretable results, leading the way to analyse smaller feature datasets in order to do counterfactual analysis targeting one variable due to intervention on another and a tracking system to recalibrate AI thresholds.
Banking
AI algorithms analyse large amounts of data and identify potential risks, such as credit default or market fluctuations, to enhance risk management strategies. AI helps with regulatory requirements by automating compliance processes and identifying potential violations (BASEL III)[xxxv]. AI can be used for loan underwriting and credit risk by analysing customer data to determine the likelihood of loan default. The AI algorithm can make decisions without prejudice if it has been trained with unbiased datasets and tested for programming bias. This contributes to fairer decisions regarding hiring, sanctioning loans, and approving credit.. The use of machine learning for credit risk modelling transformation is widespread across banks with positive results.
Stock Markets
Boardrooms need to be in control of digital transformation, cybersecurity and understand the value of data as an intangible asset and stay abreast of exponential technologies. MIT’s Sloan School of Management[xxxvi] provide insights on creating digitally savvy boards[xxxvii]. AI enabled insight is of increasing importance for boards to bring them closer to investors and this has positive implication for stock exchanges who get guidance on data driven decision making, especially on quarterly board meetings in communication with stakeholders, investors, asset owners and regulators. A poster child for AI dashboard enabled analytics is Ainstein AI[xxxviii] who currently handle underlying data to thousands of global publicly listed companies providing analytics to stock exchanges, surfacing hidden patterns and risks in the data. They use third wave AI approach which uses High Frequency Research[xxxix] trading data to support a more transparent and explainable trading process by connecting data to the underlying stock, providing research at the industry level so that peer benchmarking can be done. The diagram below shows the cube containing feature sets which can be wrapped with a risk ribbon for measurable and tracking parameters for the business sector at hand.
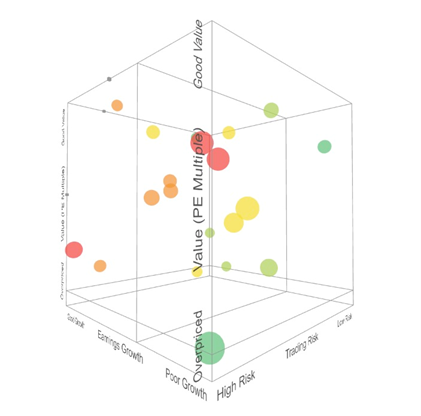
Source: Ainstein AI https://www.ainsteinai.com/investors.html
Dashboarding keeps track of all metrics, dynamically with real time data. Currently companies use a mix of manually entered data and real time data with internal and external measures of performance. Companies adopting real time dashboarding have a higher net margin than industry average and plans are to add GenAI to the analytical process in time.
Insurance and Reinsurance
AI will significantly change the insurance industry. As Generation-Z[xl] enter management and become customers, adoption of AI will be more acceptable. Knowledge of the industry captured in expert systems can be applied in third wave AI. Thousands of rating variables and questions exist, many bearing little relationship to the risk at hand so small relevant feature sets for analysis are fit for purpose. The main disruptions are underwriting, claims, fraud reduction/detection, operational efficiency and customer retention encapsulated by the way modelling is done in an AI driven world moving to predictive models. InsurTech’s and Smart MGA’s use data driven algorithms as a basis of triggers for parametric embedded insurance. AI quickly aggregates smaller feature datasets from big data lakes to accurately quantify data points and the quality of machine learning data is as vital as the quantity. It is estimated that AI can lead to $390 billion in cost savings for healthcare in USA alone by 2030[xli] as IOT is already supplying the industry with new opportunities and sources of data to analyse, Below shows the likely use cases to dominate AI in the insurance industry.
Underwriting will be automated, aided by embedded machine and deep learning models, which ingest internal/external data accessed through API’s. Information collected from devices provided by (re)insurers, product manufacturers, and distributors is aggregated into a variety of feature sets which enable insurers to make ex ante decisions regarding underwriting, pricing and bindable quotes for products tailored to the buyer’s risk profile and coverage needs. Rating engines have been widely adopted in the insurance industry in many countries for decades. These current rating engines have limitations and is why AI is now challenging them. Computer vision AI technology can assess risks associated with a physical insured asset such as a building or vehicle by utilising AI powered damage inspections assessing size and location. These computer vision programs are trained by synthetic images to increase their speed and accuracy. Internet of Things (IOT) sensors and drones are closely linked to the insurance value chain replacing traditional, manual methods of first notice of loss. IoT devices monitor key risk factors in real time and alert both insured and insurer of issues. Interventions for inspections are triggered when factors exceed AI-defined thresholds. Detailed reports automatically flow to reinsurers for faster reinsurance capital deployment. Reinsurers state that “the industry struggles to adopt new data without a clear understanding on how insights on behaviour correlate with actual risk experience”[xlii]. Third wave AI will solve that issue for reinsurers as curated and shown by Swiss Re Institute.
AI and Climate Change
Climate change is a major risk to insurance sector and how financial services in general invest without greenwashing or brown spinning. AI, underutilised in the sector, can help transition to renewable energy by integrating multiple energy sources with power grids. Use of AI algorithms do predictive analysis for more accurate anticipation of energy demand. Emerging renewable power generation companies utilise cloud-based AI weather forecasting tools to optimize power generation and avoid the fluctuation of power supply from solar and wind sources due to natural catastrophe This creates sustainable AI and directly relates to the U.N.'s sustainability goals[xliii]. As current risk modelling uses correlation (primarily regression based) this becomes a limitation as the risk increases such as spikes in 1 in 200 year floods. Modelling natural catastrophe has been mostly first wave AI and recently extended with machine learning to second wave. Such is the importance of the climate change risk plus the need for resilience, climatologists must move to the third wave and establish causal linkage with real time econometric data aggregation and pattern recognition, which increases the volume and dimensionality of the data and removes the limitations of backward looking models. Full transparency is required where the decisions are established and explained to third parties and regulators otherwise these models will become vestigial. Given the pace of technology many companies will buy and integrate AI to their current models meaning existing systems will be able to augment with causal AI rather than build it. One such company getting accurate results with AI is AbsoluteClimo[xliv]
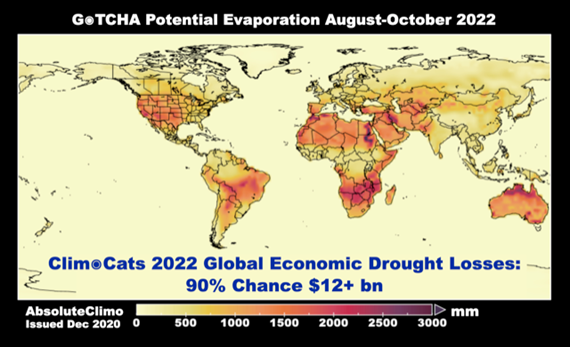
Source: AbsoluteClimo https://absoluteclimo.com/
Global energy supplies and optimising energy consumption is vital. For the electric and hydrogen vehicle market the industry still needs context-aware battery management systems that can holistically manage drawdown of battery capacity and charging. This is only possible with AI. It is expected that corporate players in the energy sector, including their supply chains, to invest and acquire AI technology. Chat-GPT was trained on a large dataset of weather data leading to weather predictions but these do not accurately reflect real-world outcomes and require integration with solutions such as AbsoluteClimo.
Enterprise AI MarketFear of missing out affects companies adding a new dynamic to overhaul legacy software to become an AI First enterprise. End-to-end machine learning vendors have combined the AI lifecycle management process into a single scalable SaaS product to build AI systems quickly and efficiently. Under this aegis multimodal AI research is powering search and content generation from a single AI model that understand video, text, and images combined. Taking projects from raw data to production AI is a multi-step process, from sourcing, cleaning data and running data quality checks and translating the whole process via natural language commands into computer code ushering in a new era for business developers who are not professional programmers and can now curate AI creation. This is not just software but a sea change in the chip industry where AI processors and memory exist on a single chip to overcome limitations of latency and privacy. These AI chips work well in autonomous devices where they need to be integrated in close proximity on cloud edge and a chip that trains a neural network faster using less energy. Photonic processors are emerging which move data at speed using light and not electricity. It is these hardware developments that will build AI supercomputers in the quantum computing era.
Real world customer data can be unusable due to being unavailable or barred by privacy regulations. Use of machine generated synthetic data is an alternative to data de-identification using simulations, machine learning or generative deep learning to create the data. Protagonists say this is the only way to address healthcare fraud detection using simulations to model causal relationships leveraging external real world entities without reversing to any real identity. Detractors say that synthetic data should never be used as it puts Enterprise AI at risk and the existence of Privacy Enhancing Technology (PET), where data does not have to be moved or shared and can automatically de-identify data keeping compliance with healthcare standards and privacy laws. A good example of a PET solution is Tripleblind[xlv]. While PET emerges and matures insurance providers will likely use a synthetic data generator while keeping the privacy of their customers intact. Insurance pricing can be improved with synthetic geodata to predict at a granular level, risk, climate data, crime statistics and publicly available databases. In call centres the transcripts of calls allow companies to detect customer dissatisfaction with sentiment analyses, intervening on potential churn thus taking pre-emptive actions and reducing costs.
Trends for Generative AI (GenAI)
GenAI shakes up the cloud computing and search engine commercial sector. The top cloud computing platforms, Amazon, Microsoft Azure, and Google Cloud, roll out specialized services on machine learning where the workloads favour the cloud’s autoscaling and pay when used model for immediate adoption on areas in demand. Image content libraries are used to train AI projects on millions of images, videos and music but royalties apply and court cases are appearing for infringing copyright by training models on data without permission. GenAI now being used in building enterprise AI in the cloud as it generates its own SaaS[xlvi] code. Microsoft is integrating Open AI into the Bing search engine increasing Bing app downloads by 800%.
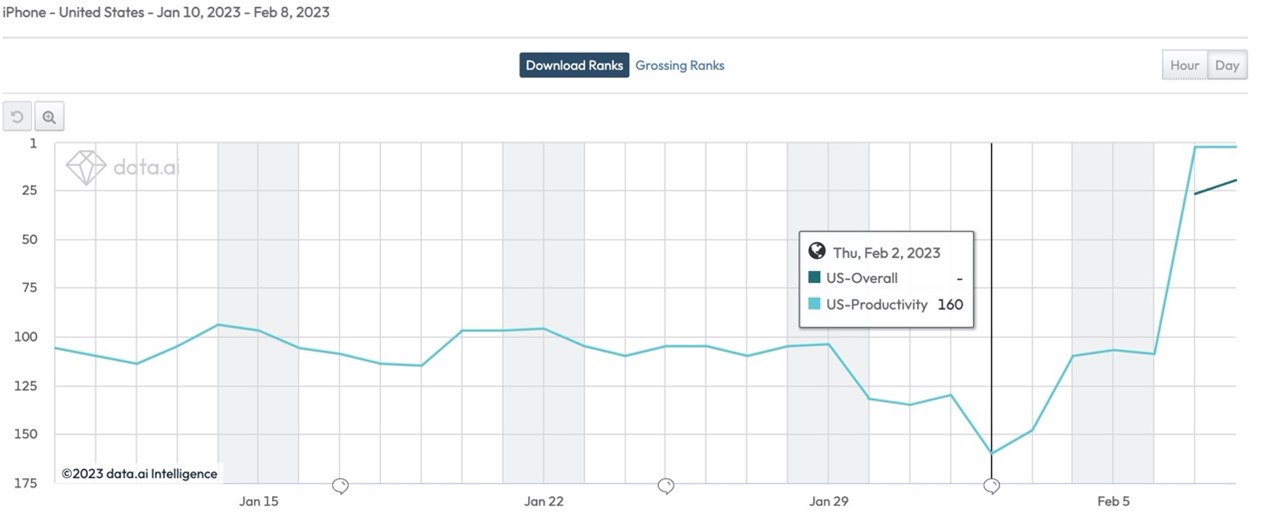
Source: https://techcrunch.com/2023/02/08/bings-previously-unpopular-app-sees-a-10x-jump-in-downloads-after-microsofts-ai-news/
China search engine Baidu has a large scale machine learning model called Ernie[xlvii] while Google is enhancing it's search engine with the Apprentice Bard[xlviii] AI chatbot. Currently Microsoft is looking at helping knowledge reuse by bringing knowledge workers together with machine learning to raise the bar for individual and collective success potential. This is a co-pilot approach to bring third wave AI and human augmentation mainstream. Amazon Web Services to date is utilising AI for internal operations.
Both Google and Microsoft are bringing LLM’s into everyday tools but there is work to do to address the source of data to avoid IP infringements. UBS calculated potential usage from around one billion knowledge workers globally with ChatGPT[xlix]. The cost of integrating and training GenAI into search results is expensive but as with exponential technology high rates of adoption costs will likely come down quickly especially if running more niche smaller sized models. Google has lower cost of adoption via its proprietary TPU chips[l].
Anthropic[li], run by former Open AI engineers, is worth a mention as deploying Claude[lii], a ChatGPT rival which has attracted Google investment of 10%[liii] and Google will also provide cloud computing services. Claude was built using the concept of constitutional AI[liv], meaning the underlying language model was trained on a “do no harm” set of principles. ChatGPT Plus is now available in USA as a chargeable version and will soon be global averaging around 13 million new visitors per day with levels doubling each month as showed by the chart. The race for the cloud, search engine and GenAI has begun at a fast pace.
Future and Conclusions
Companies should be looking at AI as human impact on business outcome not with negative thoughts about societal impact, ensuring underlying models focus on Responsible AI. 85% of AI opportunity is collaborative augmentation with human insights and 15% automation. AI systems are learning every day so this is not linear growth and must be driven by the c-suite as the risks of getting it wrong invokes a new risk register leading to reputational, financial and brand damage. HR systems with AI models can automate bias and inequality at scale so there needs to be governance and trust in these systems. Gartner predicts that as many as 85% of AI projects by the end of this decade will fail[lv]. Modelling firms should add a third wave AI layer to their models on a buy vs build basis. At WEF 2023 Microsoft CEO [lvi]identified AI as being at the beginning of an S curve signalling rapid growth. When AI is combined with other developments such a quantum computing, Web3, metaverse and developments in cloud computing and data science, the 4th Industrial Revolution has accelerated with the notation of collaboration and machine learning. The short term risk is cybersecurity, improving data integrity and generating a need to install zero trust by design and then manage the longer term risks around ethics, bias and deepfakes responsibly.
The impact on the insurance industry is significant. McKinsey have said “AI will increase productivity in insurance processes and reduce operational expenses by up to 40% by 2030”[lvii]. According to Allied Market Research, “the global AI in the insurance industry generated $2.74 billion in 2021, and is anticipated to generate $45.74 billion by 2031.”[lviii] KPMG stated “investment in AI is expected to save auto, property, life and health insurers almost US$1.3 billion”[lix]. The McKinsey Institute created a model that suggests that early adopters of AI could expect to increase cash flow by up to 122%, compared to only 10% for followers and a 23% decrease for non-adopters”[lx]
For all business sectors utilizing AI in dangerous activities can lower the risk of harm or injury to people such as robots deployment in radiation environments. However AI cannot be taken for granted once deployed as devices eventually wear out and the AI deployed can become outdated if it is not taught to learn and frequently revisited by data scientists. If the AI isn’t retrained or given the ability to learn and advance on its own, the model and training data used to generate it will eventually be out of date until AGI becomes a reality.
For financial services using AI third wave is about improving returns on the use of advanced analytics for predictive modelling clearly explaining how the AI came to decisions and keeping the human in the loop at all times except for the most mundane of tasks. Much rests on education to skill employees in the use of AI and generate new jobs on the back of the innovation. Important issues of our time such as cyber risk, climate change and pandemics cannot be addressed by humans alone hence the co-piloting with machines to insert human cognition and reasoning based on trust into financial services advanced analytics.
REFERENCES
The author would like to thank Suzanne Cook of StockSMART Aintein, Brendan Lane Larson of AbsoluteClimo and Dr Robert Whitehair and Maksim Baev of Eumonics for their input to this paper.
[i] https://analycat.com/artificial-intelligence/uncertain-accountability-in-medicine/
[ii] https://en.wikipedia.org/wiki/Monte_Carlo_method
[iii] https://openai.com/
[iv]https://the-decoder.com/stochastic-parrot-or-world-model-how-large-language-models-learn/
[v] https://www.weforum.org/events/world-economic-forum-annual-meeting-2023
[vi] https://bfsi.economictimes.indiatimes.com/news/financial-services/how-chatgpt-the-new-ai-wonder-may-transform-bfsi/97083280
[vii] https://www.dataversity.net/what-are-ai-apis-and-how-do-they-work/
[viii] https://en.wikipedia.org/wiki/Fourth_Industrial_Revolution
[ix] https://en.wikipedia.org/wiki/Post-quantum_cryptography
[x] https://www.investopedia.com/decentralized-finance-defi-5113835
[xi] https://www.bmc.com/blogs/ai-maturity-models/
[xii] https://www.nist.gov/
[xiii] https://www.vebuso.com/2018/02/idc-80-billion-connected-devices-2025-generating-180-trillion-gb-data-iot-opportunities/
[xiv] https://www.darpa.mil/
[xv] https://executive.mit.edu/course/artificial-intelligence/a056g00000URaa3AAD.html
[xvi] https://blogs.gartner.com/leinar-ramos/2022/08/10/use-causal-ai-to-go-beyond-correlation-based-prediction/
[xvii] https://www.eumonics.com/
[xviii] https://www.ediweekly.com/the-three-different-types-of-artificial-intelligence-ani-agi-and-asi/
[xix] https://research.aimultiple.com/large-language-model-training/
[xx] https://www.amazon.com/Age-I-Our-Human-Future/dp/0316273805
[xxi] https://www.wsj.com/articles/chatgpt-heralds-an-intellectual-revolution-enlightenment-artificial-intelligence-homo-technicus-technology-cognition-morality-philosophy-774331c6?mod=opinion_lead_pos5
[xxii] https://stability.ai/
[xxiii] https://aibusiness.com/nlp/ubs-chatgpt-is-the-fastest-growing-app-of-all-time
[xxiv] https://www.statista.com/outlook/dmo/fintech/digital-investment/robo-advisors/worldwide
[xxv] http://ai.stanford.edu/blog/understanding-incontext/
[xxvi] https://en.wikipedia.org/wiki/RSA_(cryptosystem)
[xxvii] https://artificialintelligenceact.eu/
[xxviii] https://gdpr.eu/
[xxix] https://www.uspto.gov/sites/default/files/documents/National-Artificial-Intelligence-Initiative-Overview.pdf
[xxx] https://www.wyden.senate.gov/imo/media/doc/2022-02-03%20Algorithmic%20Accountability%20Act%20of%202022%20One-pager.pdf
[xxxi] https://www.nist.gov/
[xxxii] https://en.wikipedia.org/wiki/Falah
[xxxiii] https://www.responsible.ai/
[xxxiv] https://towardsdatascience.com/what-is-bayesian-statistics-used-for-37b91c2c257c
[xxxv] https://www.bis.org/bcbs/basel3.htm
[xxxvi] https://executive.mit.edu/course/becoming-a-more-digitally-savvy-board-member/a056g00000WV2TMAA1.html
[xxxvii] https://www.diligent.com/insights/americas-boardrooms/digitally-savvy-boards/
[xxxviii] http://www.stocksmart.com/
[xxxix] https://en.wikipedia.org/wiki/High_frequency_data
[xl] https://en.wikipedia.org/wiki/Generation_Z
[xli] https://www.businessinsurance.com/article/20230127/STORY/912355173/AI-could-save-health-system-$360-billion-Report
[xlii] https://www.swissre.com/risk-knowledge/advancing-societal-benefits-digitalisation/machine-intelligence-in-insurance.html
[xliii] https://sdgs.un.org/goals
[xliv]https://absoluteclimo.com/tier2.html
[xlv] https://tripleblind.com/
[xlvi] https://www.masterclass.com/articles/what-is-saas?campaignid=17057064710&adgroupid=138540388680&adid=594729885741&utm_term=&utm_campaign=%5BMC%5C+%7C+Search+%7C+NonBrand+%7C+Category_DSA+%7C+ROW+%7C+EN+%7C+tCPA+%7C+EG%7CPP+%7C+BRD+%7C+ROW&utm_source=google&utm_medium=cpc&utm_content=594729885741&hsa_acc=9801000675&hsa_cam=17057064710&hsa_grp=138540388680&hsa_ad=594729885741&hsa_src=g&hsa_tgt=dsa-1456167871416&hsa_kw=&hsa_mt=&hsa_net=adwords&hsa_ver=3&gclid=EAIaIQobChMI6snB5si1_QIVylVgCh149QhQEAAYAiAAEgIYcvD_BwE
[xlvii] https://asia.nikkei.com/Business/China-tech/Baidu-to-integrate-ChatGPT-style-Ernie-Bot-across-all-operations
[xlviii] https://www.cnbc.com/2023/01/31/google-testing-chatgpt-like-chatbot-apprentice-bard-with-employees.html
[xlix] https://www.barrons.com/articles/chatgpt-investors-ai-microsoft-stock-51675284609
[l] https://en.wikipedia.org/wiki/Tensor_Processing_Unit
[li] https://www.anthropic.com/
[lii] https://scale.com/blog/chatgpt-vs-claude
[liii] https://www.theverge.com/2023/2/3/23584540/google-anthropic-investment-300-million-openai-chatgpt-rival-claude
[liv] https://www.anthropic.com/constitutional.pdf
[lv] https://neurons-lab.com/blog/managed-capacity-model/
[lvi] https://www.youtube.com/watch?v=WOvKdLZDqVE
[lvii] https://www.mckinsey.com/industries/financial-services/our-insights/insurance-productivity-2030-reimagining-the-insurer-for-the-future
[lviii] https://www.alliedmarketresearch.com/ai-in-insurance-market-A11615
[lix] https://kpmg.com/xx/en/home/insights/2019/04/powering-insurance-with-ai-fs.html
[lx]https://clappform.com/posts/artificial-intelligence-and-the-future-perspective
3.2023
About the Author:
David Piesse is CRO at Cymar. David has held numerous positions in a 40-year career including Global Insurance Lead for SUN Microsystems, Asia Pacific Chairman for Unirisx, United Nations Risk Management Consultant, Canadian government roles and staring career in Lloyds of London and associated market. David is an Asia Pacific specialist having lived in Asia 30 years with educational background at the British Computer Society and the Chartered Insurance Institute.
View More Articles Like This >